AI Solutions for Climate Change: Progress and Potential
TheUntamedSociety.com
AI Education
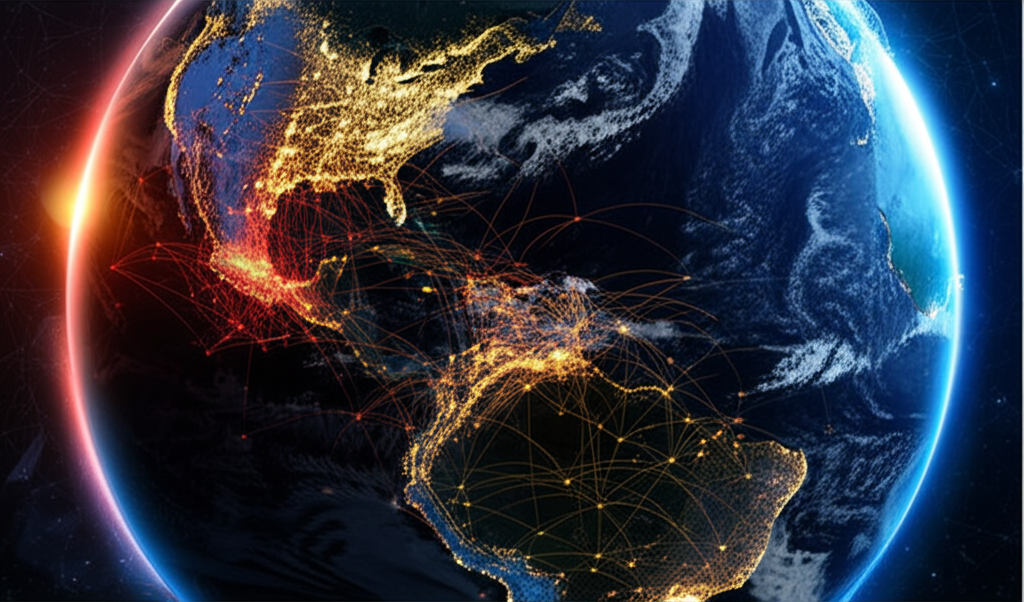
AI Solutions for Climate Change: Progress and Potential
Climate change represents one of the most urgent challenges facing humanity. As we seek solutions to reduce emissions, adapt to changing conditions, and build resilience, artificial intelligence offers powerful tools to accelerate progress. From optimizing renewable energy systems to modeling climate scenarios, AI applications are already making significant contributions to climate action.
Current Applications
AI is already being deployed across multiple domains of climate action:
Energy Systems
- Renewable Energy Forecasting: AI models predict solar and wind power generation with increasing accuracy, enabling better grid integration of intermittent renewables.
- Grid Optimization: Machine learning algorithms balance supply and demand in real-time, reducing waste and maximizing renewable utilization.
- Energy Efficiency: AI systems in buildings can reduce energy consumption by 10-20% by optimizing heating, cooling, and lighting based on occupancy patterns and weather conditions.
Environmental Monitoring
- Satellite Imagery Analysis: AI processes vast amounts of satellite data to track deforestation, monitor ice melt, and detect methane leaks from oil and gas operations.
- Biodiversity Tracking: Computer vision identifies and counts plant and animal species, helping scientists monitor ecosystem health and biodiversity loss.
- Ocean Monitoring: AI-equipped autonomous vessels and underwater drones collect data on ocean temperatures, acidification, and plastic pollution.
Climate Modeling
- Enhanced Predictions: Machine learning improves the resolution and accuracy of climate models, providing better projections of future conditions.
- Extreme Event Forecasting: AI systems help predict extreme weather events like hurricanes and floods with greater lead time and precision.
- Local Impact Assessment: Downscaled models provide community-specific climate risk assessments for adaptation planning.
Sustainable Agriculture
- Precision Farming: AI-powered systems optimize irrigation, fertilizer use, and pest management, reducing resource consumption while maintaining yields.
- Crop Monitoring: Computer vision detects plant diseases and nutrient deficiencies early, minimizing crop losses and chemical interventions.
- Supply Chain Optimization: AI reduces food waste by improving demand forecasting and distribution logistics.
Emerging Innovations
Beyond these established applications, several promising AI-powered climate solutions are emerging:
Carbon Capture and Sequestration
- Materials Discovery: AI accelerates the discovery of new materials for carbon capture by simulating molecular structures and predicting properties.
- Process Optimization: Machine learning optimizes carbon capture processes for efficiency and cost-effectiveness.
- Monitoring and Verification: AI systems track carbon sequestration to ensure permanence and accurate carbon accounting.
Circular Economy
- Waste Sorting: Computer vision systems identify and sort recyclable materials with greater accuracy than traditional methods.
- Product Design: AI tools help designers create products that are easier to repair, reuse, and recycle.
- Materials Tracking: Blockchain and AI enable better tracking of materials throughout their lifecycle, facilitating recovery and reuse.
Climate Finance
- Risk Assessment: AI models evaluate climate risks for investment portfolios, encouraging capital flows toward sustainable projects.
- Impact Measurement: Machine learning helps quantify the environmental impact of investments and business activities.
- Fraud Detection: AI systems identify greenwashing and verify environmental claims, improving market integrity.
Challenges and Limitations
Despite its potential, AI for climate action faces several challenges:
Technical Challenges
- Data Gaps: Many regions lack the historical climate and environmental data needed to train effective AI models.
- Computational Costs: Training large AI models requires significant energy, potentially contributing to emissions if not powered by renewables.
- Uncertainty Quantification: Climate applications require robust uncertainty estimates, which many AI approaches struggle to provide.
Implementation Barriers
- Infrastructure Requirements: Deploying AI solutions often requires digital infrastructure that may be lacking in developing regions.
- Skills Gap: There's a shortage of professionals with expertise in both climate science and AI.
- Cost Barriers: Initial investment costs can be prohibitive, especially for solutions targeting the Global South.
Governance Issues
- Equity Concerns: Without careful design, AI climate solutions might primarily benefit wealthy regions while neglecting vulnerable communities.
- Transparency: Many AI systems function as "black boxes," making it difficult to verify their accuracy and fairness.
- Rebound Effects: Efficiency gains from AI could lead to increased consumption if not coupled with appropriate policies.
The Path Forward
To maximize AI's contribution to climate action, several priorities emerge:
Research and Development
- Interdisciplinary Collaboration: Foster partnerships between climate scientists, AI researchers, and domain experts.
- Open Data and Models: Promote sharing of climate data and pre-trained models to accelerate innovation.
- Low-Resource AI: Develop AI approaches that can function effectively with limited data and computational resources.
Policy and Governance
- Supportive Regulations: Create regulatory frameworks that encourage AI-powered climate solutions while addressing potential risks.
- Public Investment: Increase government funding for AI climate research and deployment, particularly for public goods.
- International Cooperation: Facilitate technology transfer and capacity building across borders.
Deployment and Scaling
- Local Adaptation: Customize AI solutions to local contexts and needs rather than applying one-size-fits-all approaches.
- Public-Private Partnerships: Combine public sector guidance with private sector innovation and implementation capacity.
- Community Engagement: Involve affected communities in the design and deployment of AI climate solutions.
Conclusion
Artificial intelligence alone cannot solve the climate crisis, but it represents a powerful tool in our collective response. By accelerating scientific discovery, optimizing complex systems, and enabling more effective decision-making, AI can help humanity reduce emissions while building resilience to unavoidable impacts.
The most effective applications will combine technological innovation with appropriate policies, community engagement, and attention to equity. With thoughtful development and deployment, AI can serve as a force multiplier for climate action, helping us navigate one of the defining challenges of our time.
TheUntamedSociety.com
AI Education
Visit TheUntamedSociety.com →Expert in artificial intelligence and quantum computing with a passion for making complex technologies accessible to everyone.