How AI is Transforming Healthcare Delivery and Research
MensHealthChat.com
Health AI
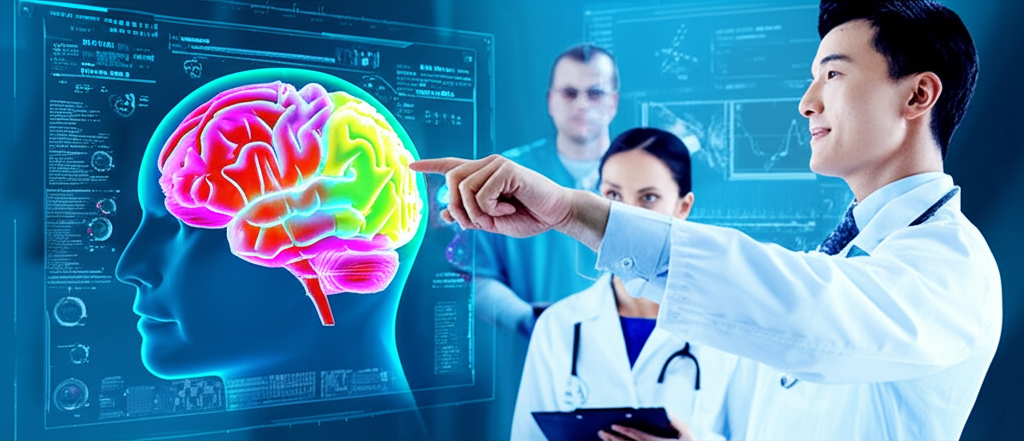
How AI is Transforming Healthcare Delivery and Research
Healthcare stands at the threshold of a profound transformation driven by artificial intelligence. From improving diagnostic accuracy to accelerating drug discovery, AI technologies are reshaping how we prevent, diagnose, and treat disease. This revolution promises not only to enhance the quality of care but also to make healthcare more accessible, personalized, and cost-effective.
Diagnostic Applications
AI is demonstrating remarkable capabilities in medical diagnosis across multiple specialties:
Medical Imaging
- Radiology: AI systems can detect subtle abnormalities in X-rays, CT scans, and MRIs, often with accuracy matching or exceeding human radiologists. Applications include identifying lung nodules, brain hemorrhages, and bone fractures.
- Pathology: Machine learning algorithms analyze tissue samples to detect cancer cells and classify tumors, providing pathologists with valuable decision support.
- Dermatology: Computer vision tools assess skin lesions and rashes, helping identify potential melanomas and other skin conditions.
Clinical Decision Support
- Symptom Analysis: AI systems evaluate patient symptoms and medical history to suggest possible diagnoses and appropriate tests.
- Risk Prediction: Machine learning models identify patients at high risk for conditions like sepsis, allowing for earlier intervention.
- Triage Optimization: AI helps prioritize emergency department cases based on severity and urgency.
Remote Monitoring
- Wearable Integration: AI algorithms analyze data from wearable devices to detect abnormal heart rhythms, blood glucose fluctuations, and other health indicators.
- Home Monitoring: Computer vision and sensor systems enable remote monitoring of elderly patients, detecting falls and changes in behavior patterns.
- Chronic Disease Management: AI-powered apps help patients manage conditions like diabetes and hypertension through personalized recommendations.
Treatment and Care Delivery
Beyond diagnosis, AI is transforming how healthcare is delivered:
Treatment Planning
- Personalized Protocols: AI systems recommend treatment plans based on patient-specific factors, including genetics, comorbidities, and previous treatment responses.
- Radiation Therapy: Machine learning optimizes radiation delivery to maximize tumor impact while minimizing damage to healthy tissue.
- Surgical Planning: AI tools help surgeons plan procedures by creating detailed 3D models and simulating outcomes.
Clinical Operations
- Resource Allocation: Predictive analytics optimize hospital staffing, bed management, and equipment utilization.
- Administrative Automation: Natural language processing extracts relevant information from medical records, reducing documentation burden on clinicians.
- Virtual Nursing Assistants: AI-powered virtual assistants handle routine patient inquiries and monitoring, freeing nurses for more complex care.
Robotics and Automation
- Surgical Robots: AI enhances robotic surgery systems, improving precision and enabling new minimally invasive procedures.
- Medication Management: Automated systems prepare and dispense medications, reducing errors and improving efficiency.
- Physical Therapy: Robotic exoskeletons and AI-guided rehabilitation systems assist patients in recovery.
Research and Drug Development
AI is accelerating medical research and pharmaceutical development:
Drug Discovery
- Molecule Screening: Machine learning rapidly evaluates millions of potential drug compounds, identifying promising candidates for further testing.
- Target Identification: AI helps identify new biological targets for drug development by analyzing genetic and proteomic data.
- Repurposing Existing Drugs: Computational methods identify new applications for approved medications, shortening the path to clinical use.
Clinical Trials
- Patient Matching: AI improves clinical trial recruitment by identifying suitable candidates based on medical records and genetic profiles.
- Real-time Monitoring: Machine learning detects safety signals and treatment effects earlier in the trial process.
- Synthetic Control Arms: For some studies, AI models can generate "virtual patients" for comparison, reducing the need for placebo groups.
Medical Knowledge Management
- Literature Analysis: Natural language processing systems analyze thousands of research papers to extract insights and identify emerging trends.
- Hypothesis Generation: AI tools suggest new research hypotheses based on patterns in existing data.
- Collaborative Research: Machine learning facilitates the integration of data across research institutions, accelerating scientific discovery.
Personalized Medicine
AI is enabling a shift toward truly personalized healthcare:
Genomic Medicine
- Variant Interpretation: Machine learning helps interpret genetic variants and their clinical significance.
- Pharmacogenomics: AI predicts how patients will respond to medications based on their genetic profile.
- Disease Risk Assessment: Algorithms evaluate genetic and lifestyle factors to estimate disease risk and guide preventive measures.
Digital Twins
- Patient Modeling: Computational models simulate individual patient physiology to predict treatment responses.
- Disease Progression: AI forecasts how conditions will evolve over time for specific patients.
- Intervention Testing: Virtual patient models allow for in silico testing of interventions before actual implementation.
Behavioral Health
- Mental Health Monitoring: Natural language processing and voice analysis detect signs of depression, anxiety, and cognitive decline.
- Personalized Interventions: AI tailors behavioral health interventions to individual needs and preferences.
- Addiction Treatment: Machine learning helps identify optimal treatment approaches for substance use disorders.
Challenges and Considerations
Despite its promise, AI in healthcare faces significant challenges:
Clinical Validation
- Evidence Standards: Establishing appropriate validation standards for AI medical applications remains complex.
- Real-world Performance: Many AI systems perform well in controlled settings but struggle in diverse clinical environments.
- Integration with Workflow: AI tools must fit seamlessly into clinical workflows to achieve adoption and impact.
Ethical and Social Issues
- Bias and Fairness: AI systems may perpetuate or amplify existing healthcare disparities if trained on unrepresentative data.
- Privacy and Consent: The use of sensitive health data raises important questions about privacy, consent, and data governance.
- Human-AI Collaboration: Defining appropriate roles for AI versus human clinicians requires careful consideration.
Regulatory Landscape
- Approval Pathways: Regulatory frameworks for AI in healthcare are still evolving, creating uncertainty for developers.
- Liability Questions: When AI contributes to clinical decisions, questions arise about responsibility for errors.
- Continuous Learning Systems: Regulations must address AI systems that continue to learn and evolve after deployment.
The Future of AI in Healthcare
Looking ahead, several trends will shape AI's impact on healthcare:
Convergence of Technologies
- Multimodal Integration: Future systems will integrate data from genomics, imaging, wearables, and electronic health records for comprehensive analysis.
- Edge Computing: AI capabilities will increasingly move to point-of-care devices, enabling real-time analysis without privacy concerns of cloud processing.
- Quantum Computing: Quantum technologies may eventually solve complex biological modeling problems beyond the reach of classical computing.
Democratization of Access
- Telehealth Enhancement: AI will further empower telehealth, bringing specialized expertise to underserved areas.
- Low-resource Settings: Simplified AI tools will support healthcare delivery in regions with limited infrastructure and specialist access.
- Patient Empowerment: AI-powered health applications will give patients greater insight into and control over their health.
System Transformation
- Value-based Care: AI will facilitate the shift from volume to value by predicting outcomes and measuring quality.
- Preventive Focus: Advanced analytics will increasingly shift healthcare toward prevention rather than treatment.
- Learning Health Systems: Healthcare organizations will continuously improve through real-time data analysis and adaptation.
Conclusion
Artificial intelligence is not merely another technological tool for healthcare but a transformative force that will fundamentally reshape the field. While significant challenges remain, the potential benefits—more accurate diagnoses, personalized treatments, accelerated research, and expanded access—are profound.
The most successful implementations will view AI not as a replacement for human clinicians but as a powerful complement to human capabilities. By combining the computational power and pattern recognition of AI with the empathy, judgment, and ethical reasoning of healthcare professionals, we can create a healthcare system that is both more advanced and more humane.
As we navigate this transformation, ongoing dialogue between technologists, healthcare providers, patients, ethicists, and policymakers will be essential to ensure that AI serves the fundamental goals of medicine: to heal, to comfort, and to improve human wellbeing.
MensHealthChat.com
Health AI
Visit MensHealthChat.com →Expert in artificial intelligence and quantum computing with a passion for making complex technologies accessible to everyone.